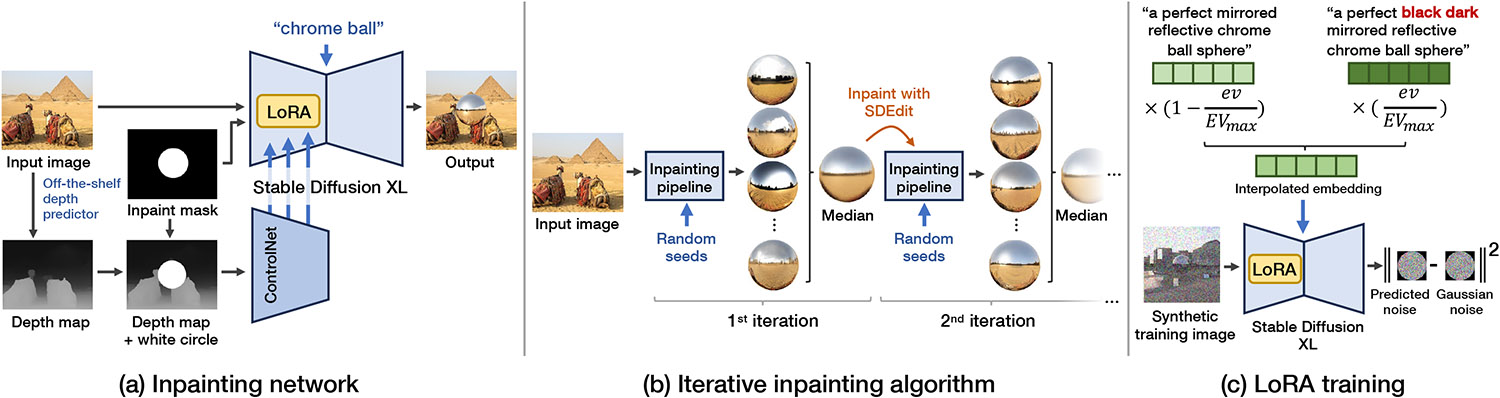
Abstract
Approach
In-the-Wild Results
Our technique can estimate lighting for a variety of input images, such as indoor and outdoor scenes, close-up shots, paintings, and photos of human faces. Here, we show our predicted chrome balls in a normally exposed version (EV0) and an underexpoed version (EV-5). These input images are from Unsplash.com.
Normally exposed
Underexposed
Normally exposed
Underexposed
Normally exposed
Underexposed
Normally exposed
Underexposed
Normally exposed
Underexposed
Normally exposed
Underexposed
Predicting Multiple Chrome Balls
Our method can generate multiple plausible chrome balls by varying the initial noise map of diffusion sampling. Note that the average of these variations captures the overall lighting reasonably well. This average is utilized by our "iterative inpainting" algorithm to enhance the quality and consistency of light estimation. Hover over these videos to pause.
Variations
Mean of the variations
Variations
Mean of the variations
Application: Virtual Object Insertion
Input image
Without relighting
Relighting with DiffusionLight
Prelim: Spatially Varying
Comparison with Prior Work
BibTex
@inproceedings{Phongthawee2023DiffusionLight, author = {Phongthawee, Pakkapon and Chinchuthakun, Worameth and Sinsunthithet, Nontaphat and Raj, Amit and Jampani, Varun and Khungurn, Pramook and Suwajanakorn, Supasorn}, title = {DiffusionLight: Light Probes for Free by Painting a Chrome Ball}, booktitle = {ArXiv}, year = {2023}, }